Behind the Scenes: How AI Really Tracks Your Calories
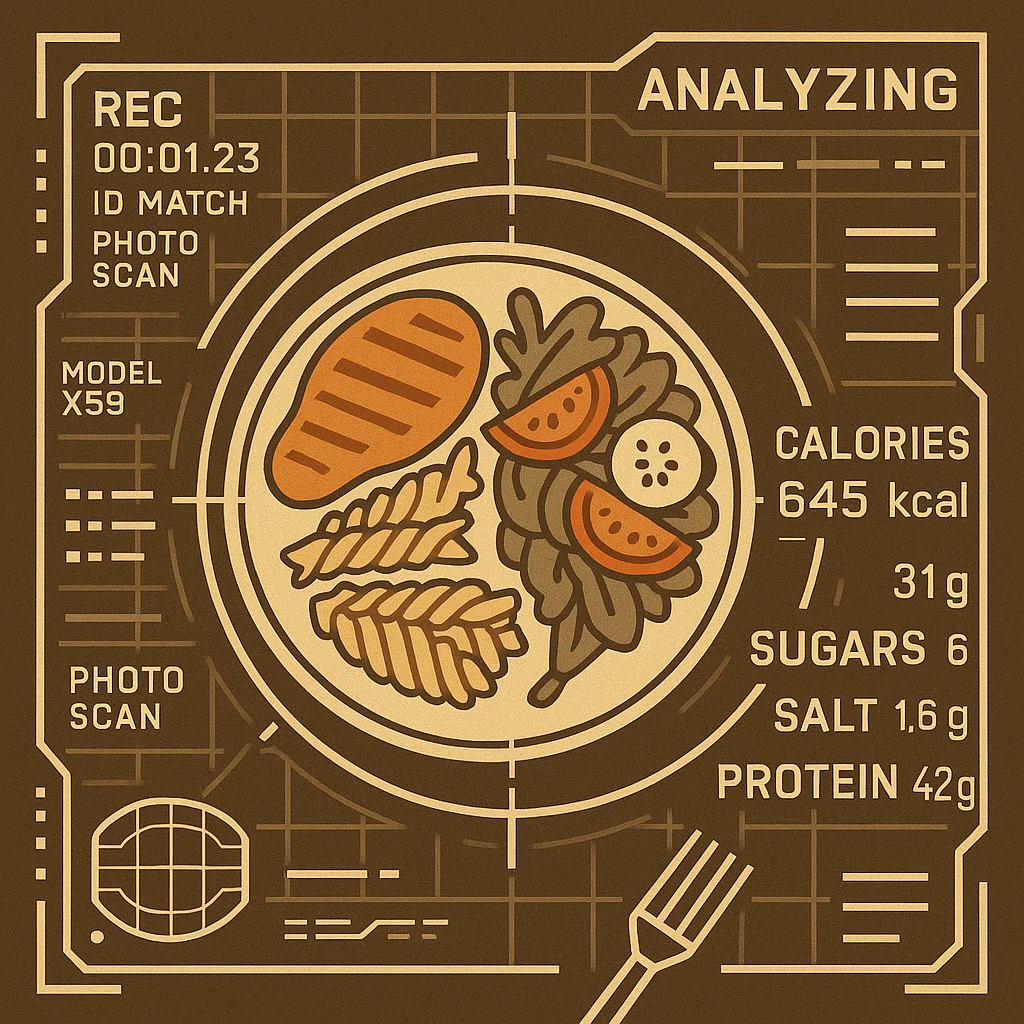
The Evolution of Food Recognition Technology
Just a few years ago, accurate food recognition from photos was considered a nearly impossible challenge. Early systems relied on simplistic computer vision techniques that could only identify a limited set of foods under ideal conditions. If lighting was poor or multiple items were on the plate, these systems would often fail completely.
The turning point came with the development of advanced multimodal large language models (LLMs) – AI systems trained on massive datasets of both text and images. These models don't just "see" your food; they understand it in context, much like a human nutritionist would.
Fun fact: Modern food recognition models are trained on millions of food images from around the world, enabling them to recognize dishes from various cuisines and cultural contexts.
How Multimodal AI "Sees" Your Food
When you take a photo of your meal, several sophisticated processes happen almost instantly:
Step 1: Image Analysis
The AI first processes the raw pixels of your photo, identifying shapes, colors, textures, and spatial relationships. This helps it distinguish between different food items on the plate.
Step 2: Object Detection
The model segments your image into regions and identifies individual food items, even when they overlap or are partially obscured. It can tell the difference between a burrito, a wrap, and an enchilada.
Step 3: Detail Recognition
The AI examines subtle visual cues that humans might miss – the browning pattern on bread indicating it's whole wheat, the texture of meat suggesting how it was cooked, or the sheen on vegetables hinting at oil content.
Step 4: Portion Estimation
Using spatial understanding and reference points, the AI estimates the volume and weight of each item. Modern systems can account for perspective and depth to make surprisingly accurate estimations.
Step 5: Nutritional Lookup
The identified foods are matched against comprehensive nutritional databases to determine calories, macronutrients, and micronutrients based on the estimated portions.
Step 6: Contextual Reasoning
Finally, the model uses its understanding of cooking methods, cuisine types, and common food pairings to fill in any missing information and refine its estimates.
The Power of Fuzzy Reasoning
What makes modern AI food recognition truly remarkable is its ability to handle ambiguity – what engineers call "fuzzy reasoning." Unlike traditional software that operates with rigid rules, large language models can:
- Make educated guesses when information is incomplete
- Integrate visual cues with text descriptions you provide
- Draw on contextual knowledge about typical ingredients in certain dishes
- Adjust confidence levels based on image quality and clarity
- Improve over time by learning from user feedback and corrections
This fuzzy reasoning is remarkably similar to how human experts work. A nutritionist doesn't need to chemically analyze your pasta to know it contains carbohydrates, and they can make reasonable estimates about portion sizes without weighing your plate. AI has finally reached a similar level of contextual understanding.
Traditional Computer Vision (Past)
- Limited to recognizing specific trained foods
- Struggled with mixed foods and complex dishes
- Required ideal lighting and angles
- No contextual understanding
- Binary "right or wrong" identification
Multimodal LLMs (Present)
- Recognizes virtually any food from any cuisine
- Handles complex, multi-component meals
- Works across various lighting conditions
- Understands context, preparation methods
- Makes probability-based assessments
When Text Meets Images: The Multimodal Advantage
The true breakthrough in food recognition came when AI models learned to process both images and text together – what's known as multimodal learning. This approach allows the AI to understand food in ways that were previously impossible.
For example, if you take a photo of a bowl of soup and add the comment "homemade chicken noodle," the AI doesn't just see liquid with floating objects – it understands you're eating chicken noodle soup and can apply its knowledge of typical ingredients and nutritional composition, even if some elements aren't clearly visible in the image.
This text-image integration creates what researchers call a "complementary information loop." The visual data helps disambiguate the text, and the text helps interpret ambiguous visual elements. The result is a system that's far more accurate and useful than one relying on images alone.
"The most powerful AI systems don't just see what's there – they understand what they're looking at within a rich contextual framework."
The Challenges and Limitations
While AI food recognition has made remarkable strides, it's important to understand its current limitations:
These limitations highlight why AI food recognition is best viewed as an intelligent assistant rather than an infallible authority. The most effective systems acknowledge uncertainty and allow for user input to refine their estimates.
Balancing Accuracy with Usability
The central challenge in AI food recognition isn't just achieving perfect accuracy – it's finding the right balance between precision and usability. As we discussed in our article on simplicity in calorie tracking, a system that's 99% accurate but too cumbersome to use consistently provides less value than one that's 90% accurate but fits seamlessly into your daily routine.
Modern AI designs intentionally make this tradeoff, prioritizing:
- Speed over exhaustive analysis – Results in seconds rather than minutes
- Intuitive interaction over technical precision – Simple photo input rather than complex questionnaires
- Consistency over occasional perfection – Encouraging regular tracking rather than sporadic precision
This approach acknowledges a fundamental truth: the most accurate nutrition tracker is the one you'll actually use every day.
The Future of AI Food Recognition
What's next for this rapidly evolving technology? Several exciting developments are on the horizon:
Personalized Nutritional Modeling
Future AI will learn your personal metabolism and adjust nutritional estimates based on how your body specifically responds to different foods, measured through continuous glucose monitors and other biometric devices.
Advanced 3D Volume Estimation
Emerging computer vision techniques will use depth sensing or multiple angles to create 3D models of your food, dramatically improving portion size estimates without requiring special hardware.
Nutritional Time Travel
AI systems will infer what you've eaten from photos taken hours later based on metabolic markers, allowing retroactive tracking even when you forget to log a meal in the moment.
Augmented Reality Integration
AR glasses will provide real-time nutritional information as you look at food, helping you make informed decisions before eating and eliminating the need to take photos entirely.
The Human Element Remains Essential
Despite these remarkable technological advances, the human element remains crucial in nutrition tracking. AI is best viewed as an intelligent assistant that reduces friction and provides guidance – not as a replacement for human judgment.
The most effective nutrition tracking systems of the future will combine cutting-edge AI with human expertise and intuition. They'll recognize when to offer precise estimates and when approximate values serve the user better. They'll understand that perfect tracking that causes anxiety or obsession is worse than imperfect tracking that promotes a healthy relationship with food.
In the end, AI's greatest contribution to nutrition tracking isn't just increased accuracy – it's increased accessibility. By making food logging simple enough that anyone can maintain it consistently, these technologies are democratizing nutritional awareness and helping millions of people develop healthier relationships with food.
Experience AI-powered nutrition tracking for yourself. Snap a photo of your next meal and see how accurately Crumpeat can analyze it – no measuring cups or food scale required.
Learn more about why simplicity matters in our article on why most calorie tracking apps fail due to unnecessary complexity.